Advanced Big Data Analytics-AI-powered data analytics
Harness AI to turn data into insights.
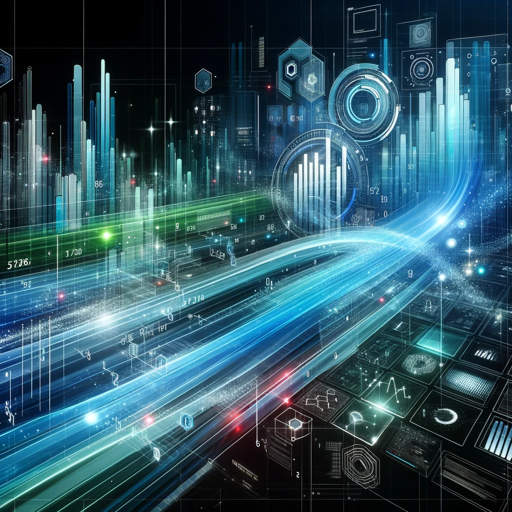
Advanced big data analysis tool for insightful patterns, trends, and predictions.
Analyze this sales data for emerging trends.
What patterns can you find in this health data?
Generate a report on recent market fluctuations.
Predict future trends based on this financial data.
Related Tools
Load More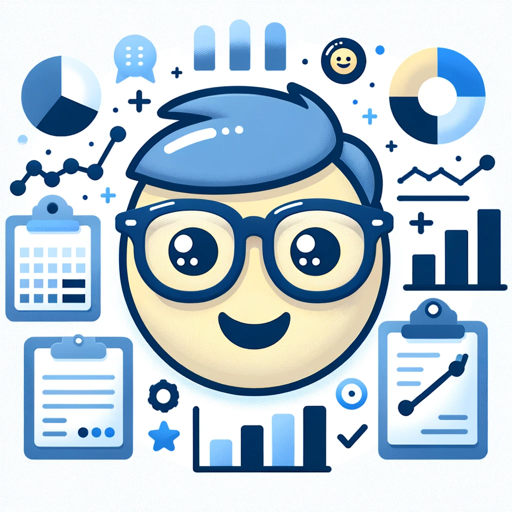
Data Analytics
A how-to guide for data analytics (based on Luke Barousse's 'ChatGPT for Data Analytics' course)

Advanced Data Analysis
Advanced data analysis assistant offering insights and guidance.
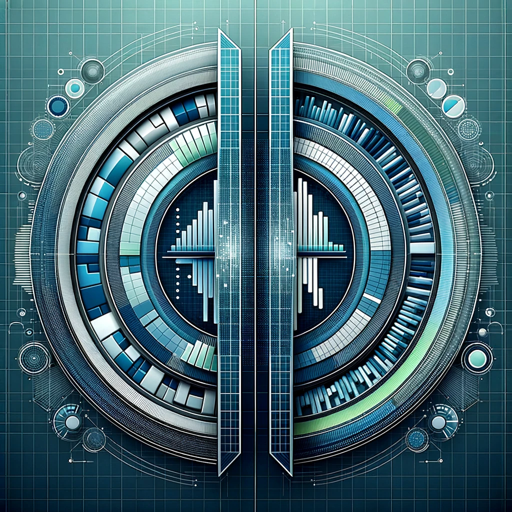
Data Science
Expert in data analysis and visualization.

Data Analyst
Data Analyst designed to guide users through data cleaning, visualization, data analysis, statistical analysis, and machine learning with practical code snippets and clear explanations

Machine Learning Expert
Expert in machine learning & artificial intelligence theory and exercises, explaining concepts with clarity and insight. Use any of these hotkeys to get a custom answer.
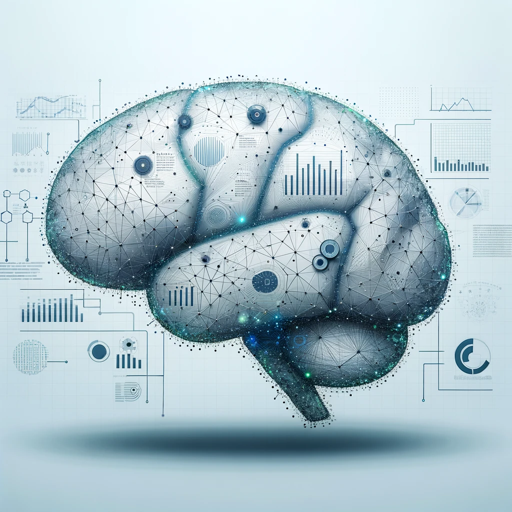
数据分析师
Data analyst with a focus on e-commerce, proficient in ML and Python. Speaks Chinese.
20.0 / 5 (200 votes)
Introduction to Advanced Big Data Analytics
Advanced Big Data Analytics is designed to process and analyze large, complex datasets to extract valuable insights, identify patterns, and drive data-driven decision-making. Its core functions focus on applying statistical models, machine learning techniques, and real-time data processing to address complex industry-specific challenges. The design purpose is to simplify and enhance the decision-making process by making high-level analytics accessible and actionable for a wide range of users, including businesses, researchers, and institutions. For example, in a marketing context, Advanced Big Data Analytics could analyze customer behavior data from millions of transactions to determine purchasing trends, allowing companies to tailor their marketing strategies in real-time. Similarly, in healthcare, it could help identify potential health risks by correlating vast amounts of patient data, leading to more effective and timely interventions.
Key Functions of Advanced Big Data Analytics
Predictive Analytics
Example
A financial institution uses predictive analytics to forecast loan default risks based on historical payment data and customer profiles.
Scenario
In banking, predictive models can analyze thousands of variables such as credit history, income, and market conditions to assess the likelihood of a borrower defaulting on a loan. By deploying these insights, banks can proactively manage risk and make data-driven lending decisions.
Real-time Data Processing
Example
An e-commerce platform leverages real-time analytics to adjust prices dynamically based on user demand and competitor pricing.
Scenario
In a competitive online retail environment, companies must adjust prices quickly to remain attractive to customers. Advanced Big Data Analytics enables real-time price adjustments by continuously monitoring market trends, competitor pricing, and consumer behavior, allowing businesses to maximize revenue opportunities.
Customer Segmentation and Personalization
Example
A telecom company uses customer segmentation to offer personalized data plans based on usage patterns.
Scenario
Telecommunication companies often deal with a wide variety of customer behaviors and needs. By analyzing customer data (e.g., call frequency, data consumption), the company can create highly targeted marketing campaigns and personalized offers, improving customer retention and satisfaction.
Ideal Users of Advanced Big Data Analytics
Enterprise Businesses and Corporations
Large organizations that generate massive amounts of data daily benefit from Advanced Big Data Analytics by optimizing operations, enhancing decision-making, and driving strategic initiatives. These include sectors like finance, retail, healthcare, and manufacturing, where detailed insights lead to cost reduction, increased efficiency, and enhanced customer experiences.
Data Scientists and Analysts
Professionals who work with complex datasets can leverage these advanced analytics tools to build and validate predictive models, discover new trends, and automate analysis processes. For instance, a data scientist in the energy sector might use these tools to optimize energy distribution by predicting demand patterns based on historical and real-time data inputs.
Guidelines for Using Advanced Big Data Analytics
Visit aichatonline.org
Access a free trial without needing to log in, and there's no requirement for ChatGPT Plus. This step provides immediate access to advanced big data analytics features.
Prepare Your Data
Ensure your data is cleaned, structured, and formatted properly. This may involve removing duplicates, handling missing values, and normalizing data. Organized data enhances analysis accuracy.
Select Appropriate Tools and Techniques
Choose the right analytics tools and techniques based on your specific use case. Whether it’s predictive modeling, data visualization, or clustering, selecting the appropriate method is crucial.
Analyze Data
Utilize the platform's advanced algorithms to process and analyze your data. Pay attention to trends, patterns, and anomalies that may inform your decision-making process.
Interpret and Apply Insights
Review the results generated by the analytics tools, interpret the insights, and apply them to your specific business or research objectives. Effective interpretation leads to better decisions.
Try other advanced and practical GPTs
Advanced Slides
AI-Powered Slide Generation Made Simple

Read Text Out Loud Bot
AI-Powered Text Reading for Everyone

Business Central Expert
AI-Powered Assistance for Microsoft Dynamics 365 Business Central

Engineering Drawing
AI-Powered Engineering Drawing Simplified
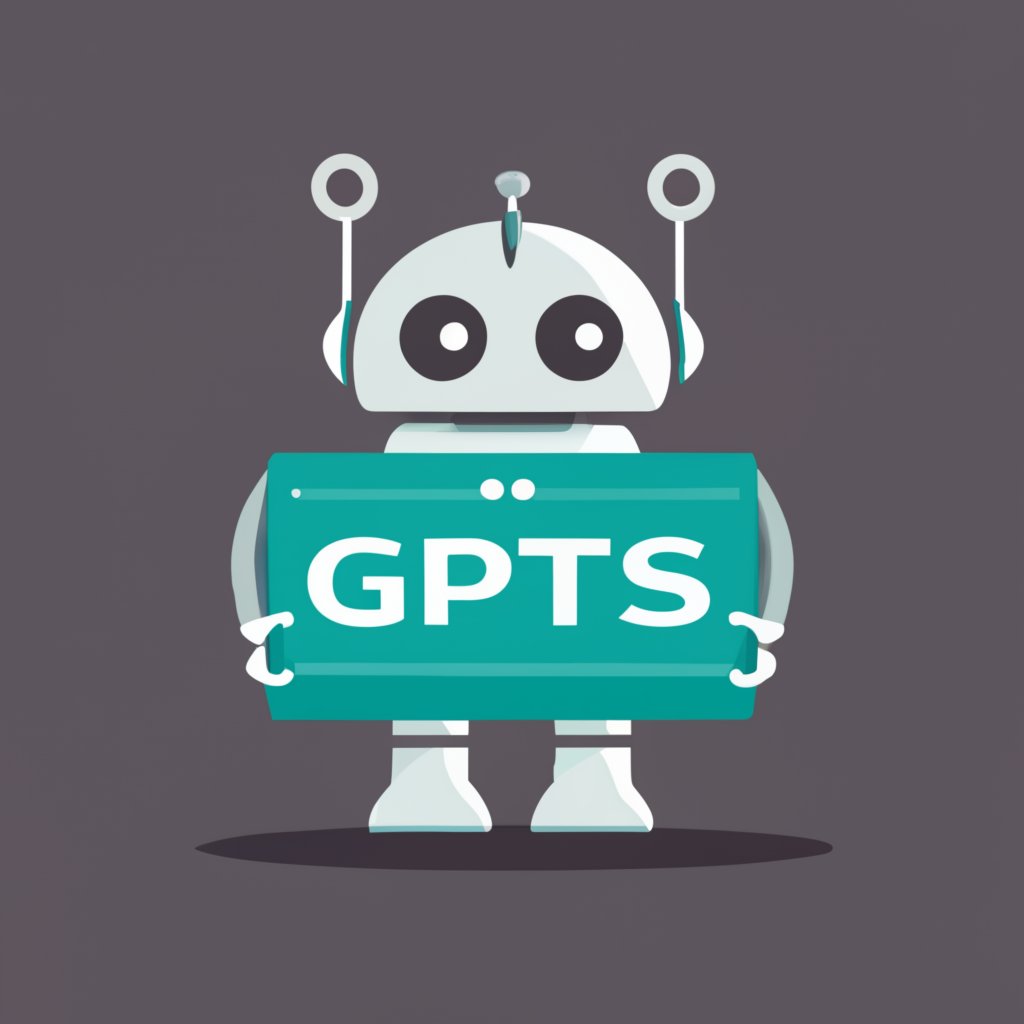
Product Requirements Document (PRD) Writer
AI-powered tool for effortless PRD creation

Functional Requirements Writer
AI-powered tool for precise requirements

Advanced SEO Writing Assistant
AI-powered tool for optimized content creation

Advanced Math and Matrix Expert
AI-Powered Advanced Math Solutions.

Advanced Physical Chemistry Tutor
AI-powered in-depth physical chemistry tutoring

Advanced Data Analysis
AI-driven insights for your data.

Advanced Heat Transfer Tutor
AI-powered insights for advanced heat transfer solutions.
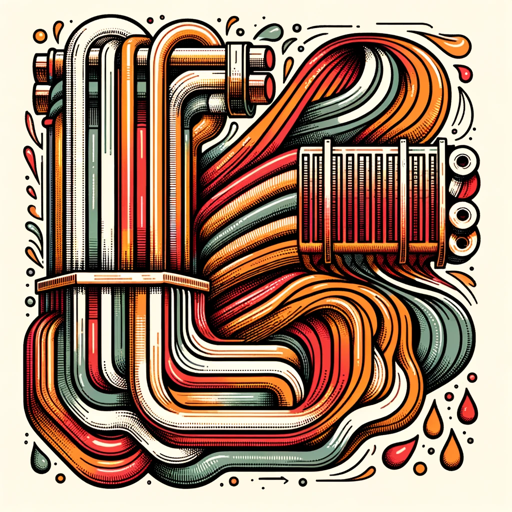
Software Testing Assistant [Manual/Automation]
AI-powered testing for seamless software quality.
![Software Testing Assistant [Manual/Automation]](https://files.oaiusercontent.com/file-MlYx58XvLrh7G8RFtIM8SNTn?se=2123-12-26T08%3A59%3A12Z&sp=r&sv=2021-08-06&sr=b&rscc=max-age%3D1209600%2C%20immutable&rscd=attachment%3B%20filename%3Dad.png&sig=w6C5QHYeFxCuVqjVv0R2TmlWio9JklIb0B5ozQvBOrQ%3D)
- Trend Analysis
- Predictive Modeling
- Process Optimization
- Customer Insights
- Data Mining
Q&A: Understanding Advanced Big Data Analytics
What is Advanced Big Data Analytics?
Advanced Big Data Analytics refers to sophisticated methods and tools used to analyze large and complex datasets, uncovering hidden patterns, correlations, and trends. It involves the use of techniques such as machine learning, predictive analytics, and data mining.
What are the common use cases for Advanced Big Data Analytics?
Common use cases include predictive maintenance in manufacturing, customer segmentation in marketing, fraud detection in finance, personalized treatment plans in healthcare, and optimizing supply chains in logistics.
What are the prerequisites for using Advanced Big Data Analytics?
Key prerequisites include access to quality data, a clear understanding of your business objectives, familiarity with analytical tools, and a basic knowledge of data processing techniques. Additionally, cloud-based platforms can simplify the setup.
How can Advanced Big Data Analytics benefit my business?
It can enhance decision-making by providing actionable insights, improve operational efficiency by identifying bottlenecks, increase revenue through targeted marketing strategies, and reduce risks by predicting potential issues before they occur.
What challenges might I face when using Advanced Big Data Analytics?
Challenges include handling data privacy and security, managing the complexity of large datasets, integrating data from multiple sources, ensuring data quality, and the need for skilled personnel to interpret and act on analytics outcomes.